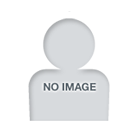
YOSHIDA TOSHINOBU
Emeritus Professor etc. | Emeritus Professor |
Researcher Information
Field Of Study
Research Activity Information
Paper
- Two-stage explicit Runge-Kutta type methods using derivatives
H Ono; T Yoshida
JAPAN JOURNAL OF INDUSTRIAL AND APPLIED MATHEMATICS, KINOKUNIYA CO LTD, 21, 3, 361-374, Oct. 2004, Peer-reviwed, Two-stage explicit Runge-Kutta type methods using derivatives for the system y'(t) = f(y(t)), y(t(0)) = y(0) are considered. Derivatives in the first stage have the standard form, but in the second stage, they have the form included in the limiting formula. The kthorder Taylor series method uses derivatives f', f'' ,..., f((k-1)). Though the values of derivatives can be easily obtained by using automatic differentiation, the cost increases proportional to square of the order of differentiation. Two-stage methods considered here use the derivatives up to f((k-3)) in the first stage and f, f' in the second stage. They can achieve kth-order accuracy and construct embedded formula for the error estimation.
Scientific journal, English - Two Stage Explicit Runge-Kutta Type Method Using Second and Third Derivatives
Toshinobu Yoshida; Harumi Ono
Journal of Information Processing Society of Japan, 44, 1, 82-87, Jan. 2003, Peer-reviwed
Scientific journal, English - Rapid Learning Method for Multilayered Neural Networks Using Two-Dimensional Conjugate Gradient Search
Toshinobu Yoshida
Journal of Information Processing Society of Japan, Information Processing Society of Japan (IPSJ), 15, 1, 79-86, 1992, Back-propagation learning in multilayered neural networks is based on the principle of steepest descent This method calculates the gradient of the error function in the reverse mode of automatic differentiation. The present paper first summarizes automatic differentiation and two-dimensional conjugate gradient search based on automatic differentiation. A new learning method using two-dimensionl conjugate gradient search is then proposed for neural networks. The proposed method automatically controls the learning rate and the momentum factor of the back-propagation. It requires computation of the quadratic forms of the Hessian of the error function. The computation time and the memory storage are proportional to the square of the size of the neural net-work if all the components of the Hessian are Computed. However, in the forward mode of automatic differentiation, the quadratic forms are computed at a cost proportional only to the size of the neural network. Numerical experiments show that the number of iterations is much smaller than for back-propagation, while the time taken for one iteration is about three times that in back-propagation.
Scientific journal, English - THE JOULE-THOMSON EXPANSION COEFFICIENT BY FORMULA MANIPULATION
Y HIROSE; T KITAZAWA; T YOSHIDA
INDUSTRIAL & ENGINEERING CHEMISTRY RESEARCH, AMER CHEMICAL SOC, 29, 7, 1555-1558, Jul. 1990, Peer-reviwed
Scientific journal, English - Rapid Learning Method for a Multilayered neural Network Using a Conjugate Gradient Method with Two-Dimensional Search
吉田利信
Journal of Information Processing Society of Japan, Information Processing Society of Japan (IPSJ), 31, 7, 988-995, 1990, Peer-reviwed, 多層神経回路網の結合係数を学習する逆伝播学習法は自助微分法の一種である.本論文では 自動微分法および自動微分法を用いた2次元探索共役勾配法の概要が示され この2次元探索共役勾配法を用いた多層神経回路網の高速学習法が提案されている.本方法は逆伝播法の学習パラメータと慣性パラメータを自動的に制御する共役勾配法であり 最急降下法である逆伝播法よりも少ない回数の繰返しで収束する.本方法にはヘッセ行列の2次形式などの計算が含まれているため ヘッセ行列を求めてからこれらの値を計算すると 神経回路網の大きさの2乗に比例した計算領域と計算量が必要となる.しかし自動微分法を用いることにより 神経回路網の大きさに比例した計算領域と計算量でこれらの値を計算することが可能である.本論文で提案されている高速学習法は 逆伝播法の高々6倍の計算量で1回の繰返し計算をする共役勾配法である.数値実験において逆伝播法 慣性項をともなう逆伝播法 学習パラメータと慣性パラメータを逐次変化させる逆伝播法および本高速学習法を用いた実験結果が比較され 本方法を用いることによって これらの逆伝播学習法よりもかなり少ない反復回数で学習が収束し 繰返し1回あたりの計算時間が逆伝播法約倍となったことが示されている.
Scientific journal, Japanese - Automatic Derivative Derivation System
吉田利信
情報処理学会論文誌, 30, 7, 799-806, 1989, Peer-reviwed
Scientific journal, Japanese - ON PERIODIC RESPONSES GENERATED BY A DEGENERATE ANALOG NEURON MODEL WITH PERIODIC INPUTS
T YOSHIDA
BIOLOGICAL CYBERNETICS, SPRINGER VERLAG, 56, 2-3, 185-194, 1987, Peer-reviwed
Scientific journal, English - Derivation of a Computational Process for Partial Derivatives of Functions Using Transformations of a Graph
吉田利信
情報処理学会論文誌, Information Processing Society of Japan (IPSJ), 28, 11, 1112-1120, 1987, Peer-reviwed, 従来 偏導関数値を必要とする数値計算には数値微分法が用いられてきた.この方法では 一つの関数のすべての偏導関数値が 変数の数に比例する回数の関数の計算によって得られる.最近 計算グラフを用いた自動微分法が提案され 一つの関数のすべての偏導関数値が 変数の数によらずに 関数自身を計算する計算量のたかだか定数倍の計算量で求められることが示された.しかしこの自動微分法を用いても 複数の関数のすべての偏導関数値を計算する場合には 関数の数または変数の数に比例する計算量が必要になる.そこで本論文では 自動微分法よりも少ない計算量の計算過程が得られる方法 すなわち 計算グラフとは別に要素的偏導関数値を辺に持つグラフ(随伴グラフ)を導入し その随伴グラフを変形する操作を繰り返すことによって偏導関数の計算過程を順次計算グラフに追加する方法を提案する.まず 従来の自動微分法に対応する随伴グラフ上の算法を基本算法として定義し 基本算法を施したときに計算グラフに追加される計算過程の計算量を評価する.次に 随伴グラフに対する基本変形を定義し 基本算法を直接施した場合と基本変形を施してから基本算法を施した場合について 追加される計算過程の計算量を比較する.この評価を用いて 一連の基本変形を施す方法が基本算法を直接施す方法よりも少ない計算量の計算過程を導出することを示す.
Scientific journal, Japanese - ON PERIODIC RESPONSES OF A MATHEMATICAL NEURON MODEL
T YOSHIDA
BIOLOGICAL CYBERNETICS, SPRINGER VERLAG, 52, 6, 383-390, 1985, Peer-reviwed
Scientific journal, English - Response Characteristics of a Mathematical neuron Model
吉田利信
電子通信学会論文誌, 61-D, 4, 276-281, 1978, Peer-reviwed
Scientific journal, Japanese
Books and other publications
Lectures, oral presentations, etc.
- 正弦成分抽出方法を用いた調波構造の抽出
佐野泰平; 吉田利信
Poster presentation, Japanese, 2016年春季研究発表会, 日本音響学会, 桐蔭横浜大学, 正弦成分抽出方法を用いて音声の調波構造の抽出し,再合成する方法を発表した.この方法を用いることで混合音声の分離が期待できる., Domestic conference
11 Mar. 2016 - 観測信号のPDFに混合ガウス分布を用い音声信号の振幅のPDFに 一般化ガンマ分布を用いたMMSE STSA 推定器による雑音低減
三田太陽; 吉田利信
Oral presentation, Japanese, 日本音響学会2013年春季研究発表会,日本音響学会2013年春季研究発表会
Mar. 2013 - 調波時間構造テンプレートを用いた 非負値行列分解に基づく楽器音源分離
加來俊輔; 高木一幸; 吉田利信
Oral presentation, Japanese, 日本音響学会,音楽音響研究会
Dec. 2010 - マルチバンド・マルチSNR・マルチパス単語音声認識における帯域結合重みに関する検討
土屋 裕一; 高木一幸; 吉田 利信
Oral presentation, Japanese, 日本音響学会2009年春季研究発表会講演論文集
Mar. 2009 - 文発話速度に対する文節発話速度の関係の分析
山本真衣; 吉田利信
Oral presentation, Japanese, 日本音響学会,2005年秋季研究発表会
Sep. 2005 - ニューラルネットワークを用いた調音モデルパラメータに 対する伝達特性の獲得
西田雅太; 吉田利信
Oral presentation, Japanese, 日本音響学会2004年春季研究発表会講演論文集
Mar. 2004 - モーラ内での子音継続時間長制御の検討
織原直史; 吉田利信
Oral presentation, Japanese, 日本音響学会2003年秋季研究発表会講演論文集
Sep. 2003 - 局所話速の算出方法の改良
中野信幸; 吉田利信
Oral presentation, Japanese, 日本音響学会講演論文集、3-10-11
Sep. 2002 - Self-Organization of Orientation Columns by Introducing a Fluctuation of Threshold
田嶋寛之; 福島邦彦; 吉田利信
Oral presentation, Japanese, 信学技報, NC2001-175
Mar. 2002 - Additional Learnable Neocognitron
柳川勝義; 福島邦彦; 吉田利信
Oral presentation, Japanese, 信学技報, NC2001-176
Mar. 2002 - An examination of speech rate measure which describes the perceived speed of speech
谷迫孝司; 吉田利信
Oral presentation, Japanese, 日本音響学会講演論文集, 1-P-19
Mar. 2002 - Compound Accentuation Rules for Compound Nouns
佐々木啓介; 吉田利信
Oral presentation, Japanese, 信学技報, SP2001-115
Jan. 2002 - 局所話速を用いた音素継続長の制御
中野信幸; 吉田利信
Oral presentation, Japanese, 日本音響学会講演論文集Ⅰ,3-Q-2
Mar. 2001 - 調音モデルに基づく声道の音響伝達特性の算出
沢田吉伯; 神原浩三; 吉田利信
Oral presentation, Japanese, 日本音響学会講演論文集Ⅰ,3-Q-24
Mar. 2001 - 音素継続長と局所話速との関係の分析
中野信幸; 吉田利信
Oral presentation, Japanese, 日本音響学会講演論文集Ⅰ,1-Q-11
Sep. 2000 - 局所話速の分析
田中晴信; 吉田利信
Oral presentation, Japanese, 日本音響学会講演論文集Ⅰ,1-Q-13
Sep. 2000 - 自己組織型階層神経回路網による時系列の認識
池田正身; 吉田利信
Oral presentation, Japanese, 信学技報,NC99-105
Mar. 2000 - 音節の継続時間長と基本周波数の相関
坂口恒彦; 吉田利信
Oral presentation, Japanese, 信学技報,SP99-87
Oct. 1999